The AI Duo Powering Smarter Fraud Detection and Insightful Analytics
by Nishant Chaturvedi, on Apr 8, 2025 4:11:28 AM
Key takeaways from this blog:
- Knowledge Graphs presents relationships between data points to answer complex questions
- Vector Embeddings uncover hidden patterns in unstructured data like text and behavior
- In combination, they enable smarter AI systems for fraud detection, search, and decision-making
In the age of data, businesses generate ginormous quantities of data every second—whether from online customer interactions and transactions, social media posts, or medical records. The issue isn't gathering it—it's making sense of it. Legacy analytics technology usually carries some limitations, especially in trying to make sense of unstructured data or recognizing underlying patterns. That is where Vector Embeddings and Knowledge Graphs enter. As used in combination, these two technologies are revolutionizing how companies analyze data, catch fraud, and provide personalization.
Through synergizing structured relationships with strong semantic comprehension, this hybrid methodology makes AI systems smarter, context-sensitive, and flexible.
🚨 Real-World Example: Stopping Fraud in Real Time
Let’s say a bank is trying to detect fraudulent transactions:
- Knowledge Graphs connect customers, accounts, devices, and past transactions
- Vector Embeddings analyze behavior to identify anomalies
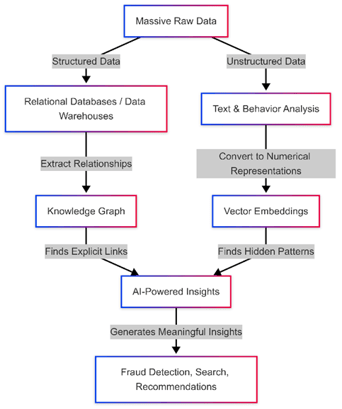
- Businesses gain a fuller picture by combining known facts (e.g., who bought what) with hidden insights (e.g., who might commit fraud next)
- This synergistic approach is already powering use cases across finance, healthcare, e-commerce, and AI-powered search engines
💡 Understanding Knowledge Graphs and Vector Embeddings
What is a Knowledge Graph?
A Knowledge Graph (KG) denotes data as a network of relationships between entities. Think of it as a web connecting customers, transactions, products, and more—not just isolated data points.
Example:
- A customer → connected to → multiple transactions
- A product → linked to → categories, suppliers, reviews
✅ Key Benefit:
Makes it easy to answer complex questions like:
“Which customers frequently purchase high-value products?”
What are Vector Embeddings?
Vector Embeddings turn complex data—like text, images, or behavior—into numerical representations. This lets AI understand similarities and patterns even when the data isn't neatly labeled.
Example Use Cases:
- Text Search: Finds documents with similar meaning, not just the same keywords
- Fraud Detection: Flags unusual behavior by comparing activity patterns
✅ Key Benefit:
Detects hidden patterns in unstructured data.
🤝 How They Work Together
When Knowledge Graphs (structured relationships) and Vector Embeddings (semantic meaning) come together, they create a powerful system that understands both what is explicitly stated and what’s hidden in the data.
🏦 Example: Smart Fraud Detection
- A bank user makes a high-value transaction
- The Knowledge Graph checks if the user is connected to any known fraud rings
- Vector Embeddings compare the user's behavior to past activity to find anomalies
- AI raises an alert if the transaction looks suspicious
✅ Why This Works:
The system goes beyond simple rules—it learns from behavior and relationships.
🔍 Application 1: Enterprise Search & AI Assistants
The Challenge
Employees struggle to find the right documents because:
- Keyword search misses context
- Documents use different terminology
- Too many irrelevant results overwhelm users
Example:
A lawyer searches for “employment contract laws” but misses key documents titled “Labor Agreement Regulations.”
✅ The AI-Powered Solution
- Knowledge Graphs map connections between legal terms, topics, and documents
- Vector Embeddings find documents with similar meaning, even with different wording
🔎 Real Example: Legal Document Search
- User searches for “Employment Contract Laws”
- Knowledge Graphs surface documents tagged with relevant legal concepts
- Vector Embeddings bring in documents with similar content
- AI ranks the most relevant ones at the top
🚀 The Impact
✅ Faster, more accurate document discovery
✅ Context-aware search
✅ Boosted organizational productivity
💳 Application 2: Fraud Detection in Banking
The Challenge
Traditional fraud systems:
- Rely on static rules
- Miss evolving fraud patterns
- Generate too many false positives
Example:
A fraud ring splits transactions across accounts to avoid suspicion—rule-based systems might miss this.
✅ The AI-Powered Solution
- Knowledge Graphs trace links between people, devices, and accounts
- Vector Embeddings detect behavior that deviates from the norm
🔎 Real Example: AI-Powered Fraud Detection
- A suspicious transaction is flagged
- Knowledge Graphs check if it’s tied to fraud networks
- Vector Embeddings evaluate if the behavior is out of character
- AI raises an alert or blocks the transaction
🚀 The Impact
✅ Real-time fraud detection
✅ Fewer false positives
✅ Adaptive defense against new fraud tactics
🔮 The Future of AI-Powered Analytics
By blending structured knowledge with semantic intelligence, businesses can unlock powerful new capabilities.
🚀 Why It Matters
- Fraud Detection: Spots both known and emerging fraud techniques
- Search & Recommendations: Understands intent, not just keywords
- Drug Discovery: Identifies hidden connections in medical data
- Real-Time AI: Continuously learns and adapts
What’s Next?
- Explainable AI: Transparent decision-making for regulated industries
- Scalable AI: Handles growing volumes of structured & unstructured data
- Collaborative AI: Provides insights that empower human decisions
🎯 Final Thoughts
The future of AI lies in blending structured data with semantic understanding. That’s exactly what Knowledge Graphs and Vector Embeddings deliver—together.
✅ Explainable – You can trust and understand AI decisions
✅ Resilient – Adapts to new data and behavior
✅ Effective – Delivers results across industries
With developing AI, companies embracing this blended approach will be at the forefront—making wiser decisions, minimizing risks, and providing improved user experiences.
🚀 The future of AI is smart, flexible, and highly contextual.
Next Reading