AI/ML, Big Data and Mobility Use Cases for Fintechs and Banks
by Smitesh Singh, on Jul 30, 2021 7:04:17 AM
With the rise of open banking, financial institutions have become the most active proponents of digitalization, disrupting the way banks work. The status quo of tech-driven business models further mandates them to embrace the machine/computer-dominated operating environment, a significant upgrade over traditional digitalization, to remain relevant to the generation of the future. Outcomes range from targeted AI/ML use cases to considerable operational efficiencies, ones made more so with the elimination of error-prone human resources. According to a recent study, the aggregate potential cost savings for banks from the application of AI in front, middle, and back offices is estimated at USD 447 billion by 2023.
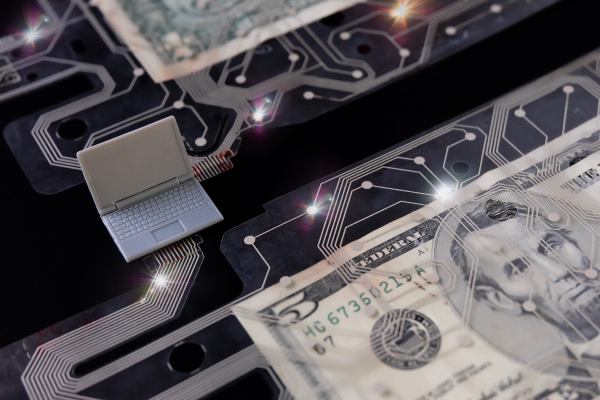
In this blog, we will talk about some popular use cases of AI and ML in the banking industry that are helping institutions battle disruption and emulate a successful business model.
Automated Customer Onboarding - Automated customer onboarding has become too mainstream to even talk about. However, consistent advances in authentication mechanisms and AI/ML-driven customer profiles have considerably helped institutions expedite customer onboarding, reduce operational costs, fuel faster execution of processes that follow, and expand the market base significantly. This is further accelerated with mobile apps, efficient and cost-effective ways to directly reach out to customers for all banking processes. Automated KYC through machine learning algorithms can also help machines become autonomous in detecting anomalies, deviations from usual behaviors as ground criteria for bigger frauds.
Credit Score Analysis - Credit score analysis offers indistinct insights on individual finances health, given that it entails the exclusion of a population who never held a credit card or borrowed a loan. Moreover, the newer insights on a customer’s browsing behavior, microloans/pay later from retail sites, and easy short-term loans from NBFCs have considerably expanded the attribute matrix for credit score analysis. Through a smartphone-based app and well-laid framework for real-time data capture and processing, you can have at hand, a substantial digital footprint of a customer, one enough to analyze the financial health of an individual.
ATM Winbacks - This is one of the AI/ML use cases recently adopted by SBI. Their AI/ML-based platform helped them identify non-SBI ATMs attracting SBI customers and identify root-cause through geospatial analytics. The satellite view of non-SBI ATMs, nearby SBI ATMs, and landmarks/businesses helped the bank streamline its presence and mark a significant reduction in ATM interchange fees in the intended areas.
NPAs Optimizations - Given the amount of data and efficiency of modern tools to extract useful insights from it, we have a strong foundation for business analysis through AI/ML-based analytics engine. These insights can be aligned and tested with the existing portfolio of NPAs, following which these assets can be further sub-categorized or written off.
Analyzing Business Prospects of Burgeoning Technologies - AI/ML and Big Data offer you a way to collect an incoming stream of high-speed data, and perform quick processing on it to derive useful insights. Data collected through feedback forms, for example, can help organizations understand the intricacies of customer satisfaction, and identify popular tools, services, UI/UX, etc. for targeted marketing campaigns. Through sales figures and related data, banks can also identify technologies that have tremendously enhanced their net worth and investments that haven’t yielded much. The banks can hence double down on strategies that work, avoiding relentless resource wastage.
Fraud and Money Laundering Detection - A machine learning platform API for fraud detection can enhance banking fraud detection by helping machines recognize frauds like email phishing, spoofing, etc., avoiding acceptable deviations from the norm including false identity, illegal access to the account, fund diversion, etc. These algorithms help machines understand historical frauds and recognize similar ones in the future. ML algorithms are able to find fraud indications that a human eye cannot. In some other cases, deviations can be flagged and may end up marked as false positive, as system feedback for “learning”. While machines perform most of the mechanical analysis, the high-level decisions still vest with officials who can control how the machines work.
Product Recommendation engine - An AI/ML-based app can help you offer personalized, popular, and ranked product recommendations to the customers through a cutting-edge collaborative filtering technique. This technique can be employed across the entire portfolio of customers generating an extra portfolio of assets such as tax-saving fixed deposits. Banks can also leverage it for a targeted selling of third-party products based on a customer’s preference.
Conclusion
The appropriation of emerging tech in banking has the potential to offer a noteworthy extent of value and enhance customer experiences through smart banking. The banks become adept at detecting dangers, monetary frauds, etc. through a clear risk possible with a customer, fostering secure choices and less credit defaulting. Hence, banks need to go beyond the inhibiting boundaries of traditional digitalization and begin a stint with AI/ML in collaboration with digital banking technology companies in order to succeed.